- eISSN 2353-8414
- Phone.: +48 22 846 00 11 ext. 249
- E-mail: minib@ilot.lukasiewicz.gov.pl
Responsible innovation in e-health care: Empowering patients with emerging technologies
Dariusz M. Trzmielak1*, Ilona Lipka-Matusiak2, Elin Oftedal3
1 Polish Mother’s Memorial Hospital Research Institute, ul. Rzgowska 281/289, 93-338 Łódź, Poland
University of Lodz, Management Faculty, ul. Matejki 22/26, 90-237 Łódź, Poland
Sukhumi State University, 61 Politkovskaya street, 0186, Tbilisi, Georgia
2 Polish Mother’s Memorial Hospital Research Institute, ul. Rzgowska 281/289, 93-338 Łódź, Poland
University of Lodz Doctoral School of Social Sciences, ul. Matejki 21/23, 90-237 Łódź, Poland
3 University of Stavanger, Institute for Media and Social Science, Kjell Arholms gate 41, 4021 Stavanger, Norway
1 E-mail: dariusz.trzmielak@iczmp.edu.pl
ORCID: 0000-0002-4455-8845
2 E-mail: ilona.lipka.matusiak@edu.uni.lodz.pl
ORCID: 0000-0003-2243-5235
3 E-mail: elin.m.oftedal@uis.no
ORCID: 0000-0002-1497-4194
DOI: 10.2478/minib-2024-0010
Abstract:
The use of new technologies should create new value for all stakeholders in the healthcare system. This article explores the transformative impact of new technologies in e-health, focusing on enhancing patient empowerment and healthcare delivery. It underscores how artificial intelligence (AI) and other innovative technologies may redefine service standards, expand functionalities, boost clinician knowledge and competencies, and relieve clinicians’ workload, thereby enabling broader and more specialized healthcare services. Through a comparative analysis of technology applications in various medical sectors, the study proposes a new, original classification of new e-health technologies according to benefits to the main healthcare stakeholders – patients, clinicians, and the healthcare system itself. The methodology includes bibliographic research and a study of selected cases of technology implementations, discussing both theoretical and practical implications for medical organizations. The classification of new e-health technologies that we propose herein a clear framework for understanding the various ways in which these innovations can be implemented to maximize their benefits across different sectors of the healthcare industry.
MINIB, 2024, Vol. 52, Issue 2
DOI: 10.2478/minib-2024-0010
P. 68-87
Published June 12, 2024
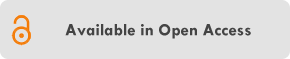
Responsible innovation in e-health care: Empowering patients with emerging technologies
Introduction
In the 21st century, the technological world is evolving with increasing rapidity. This is especially true in the field of artificial intelligence (AI), which is transforming markets in revolutionary ways. The aim of this article is to explore the impact of the development of these new AI new technologies on medical services, and products, and to classify them according to patient needs and benefits. We contribute to the literature by demonstrating the added value for the patient, for the healthcare system, and for the physicians (service providers), the interconnectedness of the factors influencing the development of new technologies, and the benefits for key stakeholders. We focus on demonstrating key innovative solutions that enable new functionalities, higher standards of service and improved clinician competence.
The article is both theoretical and practical in nature. Our primary research method is analysis of the literature and information we collected while managing the project “Implementation of a telemedicine model in the field of cardiology by ‘Polish Mother’s Memorial Hospital – Research Institute, 5/NMF/2066/00/62/2023/295, subsidized by the Norwegian Financial Mechanism and the state budget.”
First, we consider the theoretical aspects of the empowerment that emerges from new technologies, products and services, and then focus more on AI based technology for healthcare. Next, we propose an original classification of new e-health technologies according to their added value to the main healthcare stakeholders (patients, clinicians, and the healthcare system itself). Then we discuss some of the challenges faced by the implementation of new e-health technologies, products and services, and finally offer some conclusions.
Empowering new technologies, products and services — theoretical aspects
The process of harnessing new technologies and products in providing healthcare services is deeply embedded within the healthcare system as a whole. In particular, this involves healthcare providers and the services they provide, aimed at strengthening and improving the health of individuals and societies through disease prevention, early detection, treatment, and rehabilitation. Unfortunately, the healthcare situation in most European countries is expected to deteriorate due to population ageing, price increases, and the increasing complexity of healthcare technologies (Marmot et al., 2012). This entails a demand for a disproportionately high level of financial, material, and human resources.
Hence, meeting the health needs of citizens, and thus ensuring the availability of health-related services, depends primarily on a number of critical factors (Sobiech, 1990, p. 10): the volume of financial resources flowing into the health care system of a given country, the number and qualifications of medical staff, their spatial distribution and efficiency, the availability and application of medical technology and apparatus, and access to medical expertise (know-how) (Bukowska-Piestrzyńska, 2013, p. 66). With healthcare funding becoming more constrained every day, it is becoming increasingly important to look more closely at the processes of purchasing, distributing, and harnessing new technologies. Metrics such as stock coverage, urgent purchases, and non-standard purchases are particularly important in the management of healthcare facilities (Santosa et al., 2022, pp. 1-6).
The integration of AI systems in handling some aspects of communications during the diagnosis and treatment process could prove crucial for patient well-being and thus for the doctor-patient relationship. AI-based technologies, products and services will raise new questions about the limits of usability, cost-effectiveness and the ever-increasing cost of healthcare – above all, the issue of optimality in the creation of new products and services (Ayad et al., 2023).
A promising avenue of opportunities to apply new solutions to this challenge lies in digital healthcare, particularly through the implementation of artificial intelligence. These implementations involve a wide range of technologies. Digital tools are “fine-tuning” the capabilities of medical staff and facilitating a shift towards “consumerized” healthcare. This allows citizens to become more involved in managing their family’s healthcare. Digitalization, however, also brings risks, particularly if the challenges it presents are not adequately understood. If these wonderful technological advances are misused, they may expose society to the “dark side” of digital innovation. For example, if smart homes are not designed with the patient’s needs firmly in mind, but instead for the convenience of the “system,” they may give patients a prison-like experience, with robotic and sensor monitoring and control (Stahl & Cockelberg, 2016). Moreover, while AI can improve doctors’ technical skills to operate new technological solutions, it may also reduce their exposure to varied clinical experience (which in turn may make it more difficult to detect rare and atypical diseases).
Accountability in the health sector therefore raises critical questions: accountability for what, and to whom?
Ramachandran et al. (2015), for instance, reported that as many as 60% of patients with chronic diseases show interest in receiving healthcare via telephone, highlighting the a growing demand for e-health services in recent years. The management of chronic diseases is costly for individual patients and their families, as well as for the national health service. Therefore, there is great potential for developing new e-health technologies to improve the management of chronic diseases. Implementing these e-health technologies in healthcare systems can yield significant improvements and facilitate the integration of different aspects of healthcare (Hunt, 2015).
The responsible development of new technologies and bringing innovations to market require the active involvement of all stakeholders, from the very beginning of the innovation process. This helps to accurately identify the needs and priorities of innovation for society (Owen et al., 2012; Stahl et al., 2017). In health care, this means involving patients, carers and other stakeholders in the innovation process, anticipating the risks associated with new solutions, and ensuring that the solutions offered are implemented in a responsible and safe way, with the patient at the forefront (Pawelec, 2022).
New technologies and products in medicine mean smarter, safer, and more patient-centered healthcare services. By improving fit-for-purpose design, efficiency, and effectiveness, they help to reduce errors and shorten the length of hospital stays. The marketing management of healthcare services increasingly focuses on the individual purchaser – a shift emerging in many healthcare organizations thanks in part to new technologies. It should be recognized that there is an important difference between an “ordinary” customer, who can opt out of a purchase, and the patient-consumer of a healthcare service, who relies on medical consultations that directly affect his or her health or life (Białowolski et al., 2012).
Traditionally, patients have often been passive recipients at the endpoint of the service delivery system, rather than active stakeholders. One of the dangers of powerful new technologies is that patients may become even more marginalized, as healthcare is provided and delivered in an increasingly administrative, programmed manner. The doctor may also become more like a robot, carrying out programmed tasks in what could be described as “inhumane services.” The alternative approach places the patient at the center and puts technologies, products, and services at their disposal that allow them to design and control their healthcare based on their own needs. In this, it is important to shift away from seeing patients as a homogeneous group, instead categorizing them as distributed across a spectrum, including:
1)“Informed Users,” who are in a position to use technology with a better understanding;
2)“Engaged Users,” who play an activist role in the wider healthcare system, empowered by technology;
3)“Innovative Users,” who contribute their own ideas based on a deep understanding of healthcare problems.
Artificial Intelligence based technology for healthcare
Advancing safety in the organization of health technology use underscores that while consumers generally trust mature and complex technologies, advances in this area often obscure our understanding of the basics of how such technologies operate. We rely on them not because we are unaware of the potential risks, but because we believe that these risks are properly managed both by control procedures and by human oversight (by a physician). For example, we use increasingly advanced medicines without fear, often without fully grasping the complex clinical trial process that validates their safety. Similarly, we consent to robotic surgeries without fear that our health will be compromised (Turpin et. al., 2020).
The application of new technologies in healthcare should create new value, which may vary depending on the stakeholder. On the one hand, there are private companies that develop and market a technology, product or service, offering it to patients and hospitals in exchange for payment. This technology or product usually enables new functionality, a higher standard of healthcare, or a higher level of proficiency among doctors. On the other hand, there are hospitals that seek to generate maximum value, provided that does not exceed costs. Value is created when it increases revenue, enables more patients to access services, or allows diseases to be detected more quickly, improving quality of life. Value can also be derived from adhering to new global trends, such as the use of AI (Kulkov, 2021).
Artificial intelligence (AI) in healthcare involves the deployment of advanced mathematical algorithms and computer software to analyze complex medical data. The analysis of large datasets (“big data”) makes it possible to predict the probability of particular medical events. Programs that operate with the support of AI have the ability to learn autonomously (machine learning), by harnessing the collected data and the performed analyses.
Some of the first medical applications of artificial intelligence emerged in the field of radiology. AI systems are able to automatically assimilate X-ray data from databases containing thousands of images and then use this knowledge to assess a particular case and even evaluate a patient’s skeletal age (Jankowski, 2018). Physicians from the Department of Radiology, School of Medicine, Stanford University conducted a study in which 33 patients with nonspecific or common interstitial pneumonia were enrolled. Participants were selected by radiologists with 15-year experience. The same group of patients was qualified by n AI algorithm and two medics who had attended a one-year training course in the field. The AUC (area under the curve) obtained by the AI was 0.81, indicating its strong diagnostic ability. Interestingly, different diagnostic errors were found between the trained doctors and the algorithm, involving different patients. Such findings suggest the possibility of diminishing the risk associated with human error and the possibility of AI collaborating with physicians to further minimize incorrect diagnoses (Depeursinge et al, 2015).
Artificial intelligence in the field of radiology facilitates the search and analysis process for lesions, and is additionally able to detect the smallest lesions that may have been overlooked by experts (Arbabshirani et al., 2018). Recent studies also show that deep learning can adaptively improve image reconstruction during MRI examinations, leading to shorter scan times and increased quality of the obtained images, and thereby to a higher diagnostic value of the examination performed. Such improvements are particularly notable in images obtained with the FLAIR (fluid-attenuated inversion recovery) MRI sequence, which is commonly used for imaging specific brain structures (Hagiwara et. al., 2019).
A significant advantage of AI in healthcare is its potential to relieve doctors of many of their duties, allowing for more patients to be examined. An example of such an application is a study conducted on 154 diabetic patients, which investigated the efficacy of diabetic retinopathy detection based on ocular fundus examinations by the Remidio NM FOP 10, an AI-based device. Results showed concurrence in 85 cases between the device’s assessments and those of ophthalmologists. There were four instances where diabetic retinopathy lesions were identified and 81 cases with no lesions detected. Discrepancies arose in 21 cases, involving poor-quality images. The study revealed that the Remidio NM FOP 10 has a detection accuracy of 80.2%. Additionally, the device can be operated by a trained individual without an ophthalmologist’s direct involvement, potentially increasing the accessibility of preventive measures for individuals with diabetes (Kaczmarek, 2021). Deep learning holds promise for the automatic detection of diabetic retinopathy, offering consistency and precision due to its methodological approach and detailed analysis capabilities.
Another example of the application of intelligent algorithms is their use in supporting Czech medical unit doctors during appointments with specialists. Here, the AI system listens to the patient and the doctor during the appointment at the medical facility and then files a transcription of their dialog. After a few seconds, the AI generates a report from the visit, capturing the most important information provided by the patient as well as the diagnosis, recommendations, and treatment suggested by the doctor. The specialist can edit the report, add or remove specific information that the algorithm has generated. This process not only improves the visit but also allows for detailed review of previous visits, increasing the potential for seeing more patients and reducing their waiting time.
The methodologies described above have not yet been implemented in standard use. Many systems are still in the testing and observation stage in order to verify their correct functioning. Nevertheless, intelligent algorithms often yield results that are on par with, or sometimes even better than, those achieved by medical experts. The cooperation of AI systems and medical experts can minimize the risk of human error when making a diagnosis. Nevertheless, despite the attractive solutions that AI offers, there are several challenges that cannot be overlooked. It is crucial to collect, store and share medical data correctly, in accordance with current regulations. Intelligent algorithms are trained based on huge databases, with content of quality that can be difficult to access. The more information AI assimilates, the more precise the final results and diagnoses will be. Ultimately, it is crucial for results generated by AI to be verified and approved by experts in the relevant medical field (Char et al., 2018).
During the COVID-19 pandemic, new technology played an important role in allowing health services to function through increased Internet capabilities. Telemedicine, in particular, has seen significant advancements, catalyzing dynamic changes in the medical field. In addition, a variety of applications have been developed to facilitate the monitoring of patient health, as well as websites providing necessary information for those interested in such innovations. Some of the solutions are developing globally, making it possible not only to treat, but also to improve procedures or save patients’ lives, thus raising the standard of medical care in the healthcare sector.
A classification of new e-health technologies according to benefits to the main healthcare stakeholders
This section of the article explores the emerging importance of these and other cutting-edge technologies and products in healthcare. The multifaceted nature of such technologies, exhibiting high complexity, mean that a broad range of traditional health care stakeholders must be taken into consideration in the analysis of their implementation. We evaluate the benefits and added value for various groups, including medical institutions, physicians, nurses, medical technicians, distributors, e-health providers, e-health systems managers, and patients. The needs of these stakeholders vary, necessitating tailored solutions that cater to specific requirements.
While some stakeholders are involved in R&D on new technology and products, others function primarily as distributors or supporters, while still others are end-users. The literature on this topic offers various classifications of new technologies and products – notably including Herrmann et al.’s (2018) classification of over 400 different digital health projects and solutions. These were categorized according to their purpose into ten different types: software as a medical device, advanced analytics, artificial intelligence, cloud services, cybersecurity, interoperability, medical devices data systems, mobile medical applications, wireless technologies, and novel digital health solutions. However, this classification primarily focuses on products aimed at healthcare professionals, mitting those designed for the industry, the insurance companies and other stakeholders.
Severika and Ceranic (2020), in contrast, offer a broader classification of new technologies pertaining to healthcare professionals, industries, insurance companies and other stakeholders. Their proposed categories include: lifestyle intervention tools, diagnostics and prevention tools, research and development & production optimization tools, remote tracing tools, clinical decision support tools, telemedicine tools, and workflow tools. The World Health Organization (2018) emphasizes that digital and mobile technologies are increasingly crucial in supporting the needs of health systems.
From a market perspective, new technologies and products that are implemented in medical units should first and foremost add value for patients and physicians. (The economic value of new technologies cannot be overlooked, of course, but it is not the focus of this article.) From this perspective of the added value for clinicians, we propose to segment the new technologies and products into seven categories: wearable devices, mobile applications, remote monitoring systems, technologies based on artificial intelligence algorithms, telemedicine platforms, electronic health records, and 3D printing technologies. These categories underscore the specialized development and implementation needs within medicine and their potential to offer significant value to clinicians and patients. In many cases, technologies and products span multiple categories.
A detailed table of benefits for patients, doctors and the healthcare system is presented in Table 1.
We will illustrate our classification further by providing a few examples of the first product group in it: wearable devices, where the use of the product by the customer has medical applications. Several solutions will be discussed to illustrate their importance for patients.
HigoSense has created a device with 5 interchangeable tips that capture images and measurements of given areas of the patient’s body and is equipped with a module for listening to breathing, etc. This allows anyone, anywhere, to collect detailed medical data of similar quality to that obtained by a doctor during direct contact with the patient in the office or during a home visit. Data collection, delivery and sharing are carried out by means of the Higo app, which also supports medical interviews, management of patient’ history, scheduling examinations and communications with the doctor. (https://higosense.com/pl/produkt/) MedApp’s CarnaLife Holo solution employs a revolutionary technology for the three-dimensional visualization of diagnostic data to assist in the planning and execution of medical procedures. The HoloLens 2 goggles, developed by Microsoft, provide the ability to view 3D holograms of anatomical structures in a real-world environment. The doctor is able to interact with the holograms, such as rotating, scaling, moving and even entering inside the anatomical structures using gestures and voice commands. The entire process is carried out without the risk of compromising sterility and without the need to cooperate with additional technical staff. The goggles are an interactive screen that can be used for procedure planning and anywhere in the operating room, or even during the procedure. (https://medapp.pl/carnalife-holo/)
telDoc presents an innovative solution related to the Virtual Medical Assistant, which, during the patient’s contact with the medical facility, provides initial, ad hoc assistance and then refers the patient to the appropriate tests and specialist. During the visit, the doctor receives the test results and the initial medical history, which has been conducted by the Virtual Assistant via voice or chat, reducing the time spent on the administrative part of the visit. In addition, the company has created a Virtual Nurse Assistant, which regularly calls patients to ask how they are feeling, collects basic information about the patient’s vital functions, asks or reminds them to take prescribed medication, suggests contacting a medic in an alarming situation, and notifies relatives of the patient’s situation. (https://www.teldoc.eu/projekty)
Nestmedic’s Pregnabit medical device is designed for remote/hybrid KTG monitoring for women from 32 weeks of pregnancy, with indications for examination or hospitalization. The system consists of a mobile KTG device and a Medical Telemonitoring Centre service, where the test results are analyzed by medical experts. The use of specially developed medical algorithms aids doctors and midwives in monitoring and decision-making. (https://nestmedic.com/pregnabit/)
For patients, the main advantages of using modern technology of this sort include reduced access time to the doctor, increased intensity of treatment, a higher level of care resulting in better treatment outcomes, a better standard of living with chronic diseases with all-day health monitoring. Doctors, in turn, can optimize medical care and have faster, often immediate access to data in the form of epics or images. Artificial intelligence is also entering the operating theatre to assist the doctor, making procedures easier and reducing the number of repeat operations. For the healthcare system, however, it is the cost implications that are important. Estimation of the real costs of new implementations, reduction of unit costs with an increase in the number of interventions, possibility of detection of new diseases (including rare diseases).
Challenges for the implementation of new e-health technologies, products and services
Some of the key challenges include:
- Data accuracy and reliability: The accuracy and reliability of data collected by medical devices incorporating intelligent technologies is critical to the effective and efficient management of healthcare services. Patient accountability must ensure the timeliness, accuracy, relevance, appropriateness and consistency of measurements provided by AI devices (Etemadi & Khashei, 2020).
- Data security and privacy: Smart technologies generate and transmit sensitive health data, raising concerns about data security and privacy. Protecting personal data and health information from unauthorized access, breaches and misuse is paramount in the development of cyberhealth. Security measures must be implemented to protect patient data. These must be in line with data protection regulations and encryption techniques (Fatima & Colombo-Palacios, 2018).
- Integration: Integration of different smart healthcare technologies and systems is essential for seamless data exchange and collaboration (Shah et al., 2021). However, the challenges of integrating different devices, digital platforms and electronic health record systems can hinder effective data sharing and communication between patients and their doctors or healthcare organizations. One solution to this involves standardization.
- Adaptability: Devices, systems and platforms must be adapted to the type of patient, the level of health care reference, and the level of technological development of the organization implementing the new solutions (Chronaki et al., 2004).
- User acceptance and involvement: The success of the implementation of intelligent technologies depends on user acceptance and involvement. Patients need to be motivated to make consistent use of these technologies and to take an active part in their own care (Jankowska-Polańska et al., 2014). Clinicians need to follow protocols and monitor the activity and accuracy of patients’ use of the technologies. Overcoming barriers such as technology familiarity, usability concerns and resistance to change is key to widespread use of digital technologies.
- Legislation and regulation: Regulatory changes need to keep pace with the rapid development of smart technologies. Legislation needs to be put in place to ensure the safe, effective and ethical use of technology in healthcare, particularly artificial intelligence. In addition, reimbursement policies should take into account the value and cost-effectiveness of smart technologies, as this may have an impact on their availability and adoption (Orędziak, 2018).
- Accessibility: Ensuring equal access to smart technologies is essential to address inequalities in healthcare. Price, usability and accessibility of new technologies need to be considered (Bokolo, 2021).
- Validation: Rigorous clinical trials are needed for smart technologies, especially those based on artificial intelligence. Rigorous scientific research, randomized controlled trials and analysis of real-world data are needed to demonstrate the clinical value and safety of using smart technologies in healthcare. The margin for error in the use of new technologies in medicine, for example, is very small or may not exist at all. This has to do not only with protecting health, but also with protecting life (ICH Guidelines, 2016).
- Cost-effectiveness: Cost-effectiveness is an important factor in the introduction of new medical technologies. However, its role in improving quality of life and standards of care should also be emphasized (Trzmielak, 2014).
Conclusions
In the coming years, medical professionals can expect to be able to access more advanced and highly specialized tools will be available to medical professionals, increasing their competence and capabilities. Continued advances in artificial intelligence (AI) research in medicine are also likely contribute to the thorough validation of both existing and future systems, which could lead to their widespread adoption. However, the integration of advanced technologies, particularly AI, into healthcare practices represents a significant paradigm shift towards improving patient care and enhancing healthcare delivery systems. Throughout this article, we have explored the multifaceted impact of these technologies, demonstrating how they not only augment clinical practices but also empower patients by offering more personalized and accessible healthcare solutions. The bibliographic analysis and examples discussed herein offer a certain overview of the practical applications and theoretical implications of AI in healthcare, emphasizing the dual benefit to both clinicians and patients.
Our findings illustrate that AI-driven tools can significantly relieve the workload of healthcare professionals, allowing for the expansion of healthcare services and specializations that cater more directly to patient needs. This not only improves the efficiency of healthcare delivery but also enhances the quality of patient care by enabling more accurate diagnoses and tailored treatment plans. The classification of new e-health technologies that we have proposed herein may serve as a clear framework for understanding the various ways in which these innovations can be implemented to maximize their benefits across different sectors of the healthcare industry.
Moving forward, the continuous advancement and deployment of these technologies necessitates a committed approach to research and validation, ensuring that they meet the highest standards of efficacy and safety. The collaborative acceptance by healthcare professionals and patients is crucial for these technological innovations to be successfully integrated into everyday medical practices. Such acceptance is dependent on clear demonstrations of the improvements these technologies bring to patient outcomes and healthcare workflows.
In conclusion, the successful deployment of AI and other innovative technologies in medicine requires ongoing analysis and adaptation to the evolving needs of the healthcare sector. By aligning these technological advancements with the real-world requirements of both healthcare providers and recipients, we can ensure that they lead to more effective, efficient, and empathetic healthcare services. The promising developments discussed in this article not only highlight the current achievements but also pave the way for future innovations that will continue to transform healthcare.
Aknowledgements
The article was funded by the project entitled “Implementation of a telemedicine model in the field of cardiology by Polish Mother’s Memorial Hospital – Research Institute subsidized by the Norwegian Financial Mechanism and the state budget,” under contract no. 5/NMF/2066/00/62/2023/295.
References
Arbabshirani, M. R., Fornwalt, B. K., Mongelluzzo, G. J., Suever, J. D., Geise, B. D., Patel, A. A., & Moore, G. J. (2018). Advanced machine learning in action: Identification of intracranial hemorrhage on computed tomography scans of the head with clinical workflow integration. npj Digital Medicine, 1, 9. https://doi.org/10.1038/s41746-017-0015-z
Auwal, F. I., Copeland, C., Clark, E. J., Naraynassamy, C., & McClelland, G. R. (2023, September). A systematic review of models of patient engagement in the development and life cycle management of medicines. Drug Discovery Today, 28(9), 103702. https://doi.org/10.1016/j.drudis.2023.103702
Ayad, N., Schwendicke, F., Krois, J., S. van den Bosch, Bergé, S., Bohner, L., Hanisch, M., & Vinayahalingam, S. (2023, December). Patients’ perspectives on the use of artificial intelligence in dentistry: A regional survey. Head & Face Medicine, 19, Article 23. https://doi.org/10.1186/s13005-023-00368-z
Białowolski, P., Grabowska, I., Kotowska, I., Strzelecki, P. &Węziak-Białowolska, D. (2012). Social Diagnosis 2011: The objective and subjective quality of life in Poland. Ed. Czapiński, J., & Panek, T. The Council for Social Monitoring Warsaw.
Bokolo, A. Jnr. (2020). Application of telemedicine and eHealth technology for clinical services in response to the COVID-19 pandemic. Health and Technology, 11, 359–366. https://doi.org/10.1007/s12553-020-00516-4
Bukowska-Piestrzyńska, A. (2013). Zmiany w systemie opieki zdrowotnej a dostępność usług stomatologicznych w Polsce w XXI wieku [Changes in the healthcare system and the availability of dental services in Poland in the 21st century]. Vol. XIV(10), pt. I. [in Polish]
Char, D. S., Shah, N. H., & Magnus, D. (2018). Implementing machine learning in health care — Addressing ethical challenges. New England Journal of Medicine, 378(11), 981–983. https://doi.org/10.1056/NEJMp1714229
Chronaki, C. E., Lelis, P., Chiarugi, F., Trypakis, D., Moumouris, K., Stavrakis, H., Kavlentakis, G., Stathiakis, N., Tsiknakis, M., & Orphanoudakis, S. C. (2004). An open eHealth platform for health management using adaptable service profiles. International Congress Series, 1268. https://doi.org/10.1016/j.ics.2004.03.201
Depeursinge, A., Chin, A. S., Leung, A. N., Terrone, D., Bristow, M., Rosen, G., & Rubin, D. L. (2015). Automated classification of usual interstitial pneumonia using regional volumetric texture analysis in high-resolution computed tomography. Investigative Radiology 50(4), 261–267. https://doi.org/10.1097/RLI. 0000000000000127
Etemadi, S., & Khashei, M. (2020). Data accuracy and reliability. Computers in Biology and Medicine, 141. https://doi.org/10.1016/j.compbiomed.2021.105138
Fatima, A., & Colomo-Palacios, R. (2018). Security aspects in healthcare information systems: A systematic mapping. Procedia Computer Science, 138, 12–19. https://doi.org/10.1016/j.procs.2018.10.003
Hagiwara, A., Otsuka, Y., Hori, M., Tachibana, Y., Yokoyama, K., Fujita, S., Andica, C., Kamagata, K., Irie, R., Koshino, S., Maekawa, T., Chougar, L., Wada, A., Takemura, M., Hattori, N., & Aoki, S. (2019). Improving the quality of synthetic FLAIR images with deep learning using a conditional generative adversarial network for pixel-by-pixel image translation. American Journal of Neuroradiology, 40(2), 224–230.
Herrmann, M., Boehme, P., Mondritzki, T., Ehlers, J. P., Kavadias, S., Truebel, H. (2018). Digital transformation and disruption of the health care sector: Internet-based observational study. Journal of Medical Internet Research, 20, 104–112. https://doi.org/10.2196/jmir.9498 Hunt, C. W. (2015). Technology and diabetes self-management: An integrative review. World Journal of Diabetes, 6(2), 225–33. https://doi.org/10.4239/wjd.v6.i2.225
ICH Guidelines. (2016). Harmonized ICH Guidelines, Integrated Addendum to ICH E6(R1): Good Clinical Practice, E6(R2), version 4. International Council for Harmonization of Technical Requirements for Pharmaceuticals for Human Use (ICH). https://database.ich.org/sites/default/files/E6_R2_Addendum.pdf
Jankowski M., (2018). Sztuczna inteligencja jako narzędzie wspomagające proces diagnostyczno-terapeutyczny, Menadżer Zdrowia, październik – listopad 8-9, 66–67.
Jankowska-Polańska, B., Ilko, A., & Wleklik, M. (2014). Wpływ akceptacji choroby na jakość życia chorych z nadciśnieniem tętniczym [Influence of the acceptance of the disease on quality of life of patients with hypertension]. Nadciśnienie Tętnicze, 18(3), 143–150. [in Polish]
Kaczmarek, E. (2021). Sztuczna inteligencja – pomoc w wykryciu retinopatii cukrzycowej [Artificial Intelligence – Assistance in Detecting Diabetic Retinopathy]. Optyka, 6(73), 48–49. [in Polish]
Kulkov, I. (2023). Next-generation business models for artificial intelligence start-ups in the healthcare. International Journal of Entrepreneurial Behavior & Research, 29(4).
Marmot M., Allen J., Bell R., Bloomer E., Goldblatt P., (2012). WHO European review of social determinants of health and the health divide, Lancet 2012; 380, 1011–29. 10.1016/S0140-6736(12)61228-8
Orędziak, B. (2018). Telemedycyna a konstytucyjne prawo do opieki zdrowotnej w kontekście wykluczenia cyfrowego [Telemedicine and the Constitutional Right to Healthcare in the Context of Digital Exclusion]. Zeszyty Prawnicze, 18(1). https://doi.org/10.21697/zp.2018.18.1.06 [in Polish]
Owen, R., Macnaghten, Ph., Stilgoe, J., (2012). Responsible Research and Innovation: From Science in Society to Science for Society, with Society. Science and Public Policy, 39(6), 751–760. https://doi.org/10.1093/scipol/scs093.
Pawelec, G. (2022). Rola nowych technologii w podnoszeniu jakości usług zdrowotnych w dobie pandemii COVID-19. [The role of new technologies in improving the quality of health services in the era of the COVID-19 pandemic]. Marketing i Rynek, XXIX(2). https://doi.org/10.33226/1231-7853.2022.2.2 [in Polish]
Ramachandran, N., Srinivasan, M., Thekkur, P., Johnson, P., Chinnakali, P., & Naik, B. N. (2015). Mobile phone usage and willingness to receive health-related information among patients attending a chronic disease clinic in rural Puducherry, India. Journal of Diabetes Science and Technology, 9(6), 1350–1. https://doi.org/10.1177/1932296815599005
Santosa, E. S., Fariab, S. C. M., Carvalhob, M. I. S., Molc, M. P. G., Silvab, M. N., & Silva, K. R. (2022, December). Management of unused healthcare materials and medicines discarded in a Brazilian hospital from 2015 to 2019. Cleaner Waste Systems, 3. https://doi.org/10.1016/j.clwas.2022.100046
Severika, B., & Ceranic, K. (2020, April). Digital Health Classification Systems. Statistics & Science. https://www.5-ht.com/en/media/blog/digital-health-classification-systems
Stahl, B.C. and Coeckelbergh, M. (2016) Ethics of Healthcare Robotics: Towards Responsible Research and Innovation. Robotics and Autonomous Systems, 86, 152–161. https://doi.org/10.1016/j.robot.2016.08.018
Shah, J. L., Bhat, H. F., & Khan, A. I. (2021). Integration of Cloud and IoT for smart e-healthcare. In V.E. Balas, S. Pal (Eds.). Healthcare Paradigms in the Internet of Things Ecosystem (pp. 101–136). Elsevier. https://doi.org/10.1016/B978-0-12-819664-9.00006-5.
Stahl, S., Morrissette, D. A., Faedda, G. L., Fava, M., Goldberg, J., Keck, P., Lee, Y., Malhi, G., Marangoni, C., Mcelroy, S., Ostacher, M., Rosenblat, J., Solé, E. Suppes, T. Takeshima, M. Thase, M., Vieta, E., Young, A. Zimmerman, M. McIntyre, R. (2017). Guidelines for the recognition and management of mixed depression. CNS Spectrums. 22(2), 203–219. https://doi.org/10.1017/S1092852917000165.
Sobiech, J. (1990). Warunki wyboru ekonomiczno-finansowych mechanizmów kierowania opieką zdrowotną. Zeszyty Naukowe, 109. Wydawnictwo Akademii Ekonomicznej w Poznaniu.
Trzmielak, D. M. (2013). Komercjalizacja wiedzy i technologii – determinanty i strategia [Commercialization of knowledge and technology – determinants and strategy]. Łódź: Wydawnictwa Uniwersytetu Łódzkiego. [in Polish]
Turpin, R., Hoefer, E., Lewelling, J., & Baird, P. (2020). Machine Learning AI in Medical Devices, Adapting Regulatory Frameworks and Standards to Ensure Safety and Performance. AAMI, BSI. https://www.medical-device-regulation.eu/wp-content/uploads/2020/09/machine_learning_ai_in_medical_devices.pdf
Ullah, M., Hamayun, S., Wahab, A., Khan, S. U., Rehman, M. U., Haq, Z. U., Rehman, K. U., Ullah, A., Mehreen, A., Awan, U. A., Qayum, M., & Naeem, M. (2023, November). Smart Technologies used as Smart Tools in the Management of Cardiovascular Disease and their Future Perspective. Current Problems in Cardiology, 48. https://doi.org/10.1016/j.cpcardiol.2023.101922
Verbraecken, J. (2021, September). Telemedicine in Sleep-Disordered Breathing: Expanding the Horizons. Sleep Medicine Clinics, 16(3), 418–445.
World Health Organization. (2018). Classification of Digital Health Interventions v 1.0: A shared language to describe the uses of digital technology for health. https://apps.who.int/iris/bitstream/handle/10665/260480/WHO-RHR-18.06-eng.pdf
Online sources:
https://higosense.com/pl/produkt/
https://medapp.pl/carnalife-holo/
https://nestmedic.com/pregnabit/
https://www.teldoc.eu/projekty
Dariusz M. Trzmielak is Deputy Director of the Research Institute for Scientific Affairs of the Polish Mother’s Memorial Hospital and an expert in knowledge management, transfer of intellectual property from scientific and research centres to companies. He is an Associate Professor at the University of Lodz, Department of Marketing, Faculty of Management, Head of the Marketing Research Unit. He is also a visiting professor at Sukhumi State University in Tbilisi. He obtained his last degree in economics on the basis of his research work “Commercialisation of Knowledge and Technology – Stimulants and Strategies”. He worked for the American-Polish Offset Programme at the University of Texas – University of Lodz as the Director of the Innovation Centre. He was a member of the Investment Committee of the StartMoney Fund. He also managed the Innovation Centre – Technology Accelerator Foundation of the University of Lodz as Vice President of the Board of Directors. For nine years he was a member of the Board (Vice-President and Treasurer) of the Association of Organisers of Innovation and Entrepreneurship Centres in Poland and a member of the Scientific Council of the Proakademia Research and Innovation Centre.
Ilona Lipka-Matusiak – specialist in the Department of Scientific and Research Cooperation, inspector at the Centre for Support of Clinical Research in Oncology of the Polish Mother’s Memorial Health Centre Institute; teacher of economics and management. She is in the midst of an implementation doctoral programme and has received funding from the Ministry of Education and Science to implement the results of her dissertation. She was a member of the Business Creativity Circle at the Faculty of Management at the University of Lodz and cooperated with the Interdisciplinary Research Circle at the Doctoral School of Social Sciences at the University of Lodz.
Elin Merethe Oftedal is a professor at the University of Stavanger, Faculty of Social Sciences, Department of Media and Social Sciences. Previously, she was an associate professor at UiT – Norway’s Arctic University for ten years.
Her research spans entrepreneurship, innovation and sustainability, with a focus on responsible innovation, digital health and the role of education in fostering entrepreneurial intentions. Oftedal has co-authored numerous publications on these topics, exploring sustainable value creation, responsible innovation in healthcare, and the influence of university contexts on student creativity and entrepreneurial education.
In particular, her work examines the strategic role of innovation in markets, the challenges of commercialising product innovation, and the socio-emotional aspects of health technology adoption, such as promoting the use of health apps among the elderly. Her extensive contributions reflect a commitment to responsible and sustainable innovation in the context of rapid technological advancement and global change.